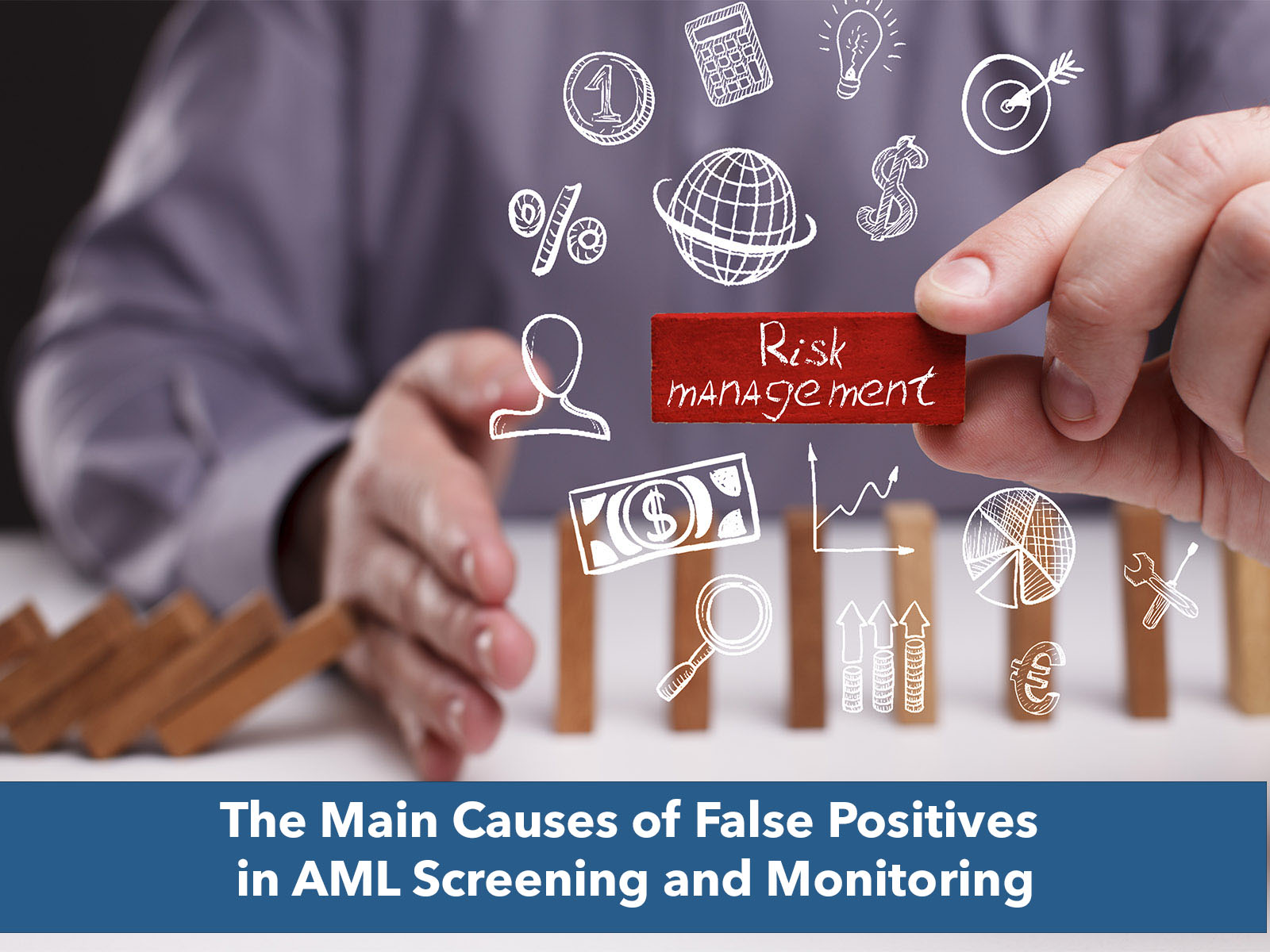
What are false positives in AML screening and AML transaction monitoring?
False positives in AML screening result when AML screening and transaction monitoring tools return results that are inaccurate, i.e. falsely positive hits. For example, when screening the name of a beneficial owner against sanctions lists, the AML screening software might return hundreds of false-positive hits.
And each hit creates a case that AML Compliance teams must resolve. In terms of efficiency and cost, false positives in AML Compliance work represent an avalanche of costly remediation work.
What tends to cause false positives in AML screening and transaction monitoring?
Many factors contribute to false positives in AML screening and AML transaction monitoring. One major factor is a foundational principle of AML Compliance. In AML Compliance there is no single set of monitoring rules, screening parameters, procedures, or way to ‘do’ AML. Instead, every institution must define and adhere to its own Risk-based Approach.
This means that no single answer or set of rules or out-of-the-box AML software solution works for any institution. Therefore, each institution must manifest its unique Risk-based approach in its AML software and desktop procedures. This represents an ongoing challenge filled with endless decision-making and adapting to new and changing regulations and AML software tools.
In this context of operationalizing a Risk-based approach, certain decisions can lead to floods of false positives. The following are examples of factors that increase false positives:
Cause: Misunderstanding algorithms in your screening software
Users often lack knowledge of how to configure algorithms in their screening systems. And sometimes they lack knowledge of the purpose and limitations of their algorithms.
For example, fuzzy logic algorithms are key to screening software, but improper configuration creates massive numbers of false positives. Users who commit themselves to knowing why and how to configure their algorithms greatly minimize false positives.
For example, users commonly misunderstand what their fuzzy-logic searches are telling them. What are the percentages of match being conveyed by your system? A 100-percent match doesn’t mean the screened name is exactly the same as the name in a list. Rather, a 100-percent match might mean the screening matched 100 percent of your criteria configured in the algorithm. If you have the algorithm set at a 75-percent-match threshold, then a screening return of 100 percent doesn’t mean the name matches 100 percent.
The challenge of understanding your algorithms increases when institutions use multiple algorithms, which is very common. Multiple algorithms used together increase the power and accuracy of AML screening software. But this holds true only if users understand the purpose and configurations for each one. Further, end-users also need to understand what happens when algorithms work together via and/or statements.
To solve this problem, you can work with your AML consultant to walk you through the algorithms in your software step by step. And repeat the learning process until you understand fully how your algorithms work. Unfortunately, this training often gets rushed or overlooked altogether. When that happens, users often make too many assumptions. Every screening system is different, and users need to understand every system in the context of their workflows and their institution’s whole Risk-based Approach.
Cause: Screening everything without regard to context
Screening everything against everything every time leads to mountains of false positives. The much more effective context-level screening requires adhering to a logical framework for screening.
For example, you screen sanctions lists against real-time transactions. In this context, you don’t care about negative news or PEP hits in real-time transaction screening. Those are lower-level risks that can be mitigated later. And in your nightly screening, you don’t want to screen your entire database of names against the entire sanctions, negative news, and PEP lists. Logic dictates that users configure nightly screening to screen only against the changes (i.e. deltas) to those lists.
Institutions that screen everything against everything all the time will be buried in false positives. In contrast, careful consideration of context-level screening will greatly reduce false positives—in line with their Risk-based Approach.
Cause: Creating rules for AML Transaction Monitoring that are not aligned to your Risk-based Approach
Most AML Transaction Monitoring software uses a rules-based approach. This means that users specify transaction behaviors that are suspicious, and the system monitors for those transaction behaviors.
Writing rules that mirror an institution’s Risk-based Approach requires deep knowledge of the institution and its customers. And it requires deep knowledge of the institution’s Risk-based approach. Writing rules that align precisely to an institution’s Risk appetite poses a lot of challenges.
Rules that are too conservative or that are poorly configured will generate a lot of false positives. But rules that don’t succeed in flagging true Risk endanger the institution in the context of AML Compliance.
As always, success with writing effective rules for transaction monitoring hinges on knowledge of the institution’s Risk-based Approach. Deep knowledge of the Risk-based Approach will improve the balance between identifying true Risk and minimizing false positives.
Cause: The pressure to comply successfully
Financial institutions can face steep penalties for inadequate AML Compliance. That pressure to achieve regulatory and AML Compliance can lead to institutions erring on the side of caution. If this manifests in overly conservative screening and monitoring choices, false positives may skyrocket.
Institutions that work to create and adhere to a carefully considered Risk-based Approach can manage the pressures of AML Compliance and GRC. With a strong Risk-based Approach and AML software solutions that mirror that approach, an institution can trust and verify that their AML decision-making aligns with their stated intent.
Solving the problem of false positives in AML
Knowing the what and why of false positives in AML helps identify solutions. In brief, a strong Risk-based Approach and high quality AML software solutions will help AML specialists greatly reduce false positives. Key technology features will enable automatic whitelisting, automatic deduplication, and automatic secondary evaluation. To learn more about those tools and how they work, check out Part 2 of our discussion about minimizing false positives in AML Compliance.